AI for Science
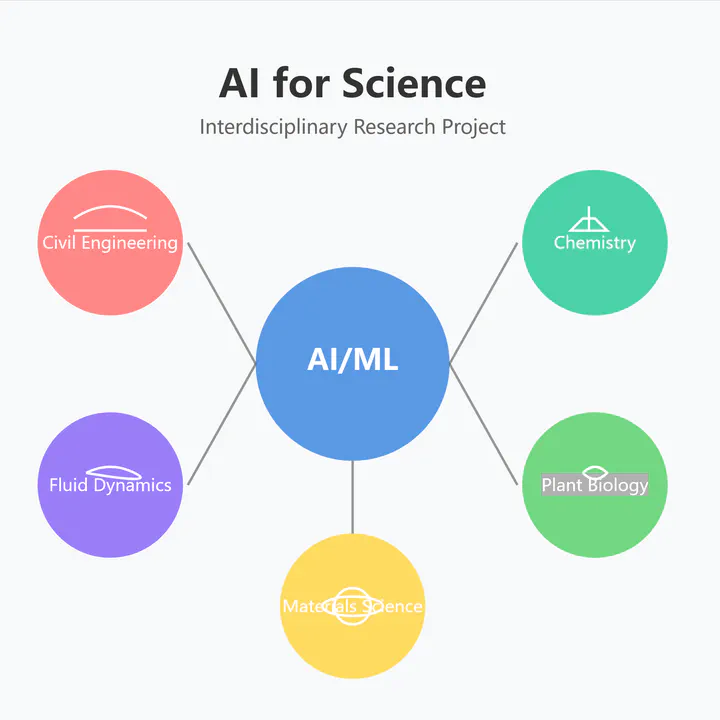
Overview
The “AI for Science” project leverages cutting-edge artificial intelligence (AI) and machine learning (ML) techniques to address complex scientific challenges across diverse domains, including civil engineering, chemistry, fluid dynamics, plant biology, and materials science. By integrating AI with domain-specific knowledge, this project aims to accelerate scientific discovery, improve predictive modeling, and enable data-driven decision-making in research and industry.
Objectives
- Develop AI-driven solutions for anomaly detection, predictive modeling, and optimization in scientific and engineering applications.
- Bridge the gap between data-driven methods and physics-based models to enhance interpretability and generalizability.
- Enable rapid and non-destructive phenotyping in biological systems.
- Accelerate computational screening of materials and catalysts for energy applications.
- Foster interdisciplinary collaboration between AI researchers and domain experts.
Methodologies
The project employs a variety of AI and ML techniques, including:
- Deep Learning: Convolutional neural networks (CNNs), Siamese networks, and physics-informed neural networks (PINNs).
- Active Learning: Accelerated computational screening and optimization.
- Data-Driven Modeling: Integration of big data analytics with domain-specific knowledge.
- Non-Destructive Techniques: Rapid phenotyping and anomaly detection.
Key Outcomes
The project has produced several high-impact publications, demonstrating the versatility and effectiveness of AI in scientific research:
-
Generalization of Anomaly Detection in Bridge Structures
- Paper: Alireza Ghiasi, Zhen Zhang, Zijie Zeng, Ching Tai Ng, Abdul Hamid Sheikh, Javen Qinfeng Shi (2025). Generalization of anomaly detection in bridge structures using a vibration�\based Siamese convolutional neural network. Computer-Aided Civil and Infrastructure Engineering.
- Outcome: Developed a vibration-based Siamese CNN for detecting structural anomalies in bridges, enabling early and accurate identification of damage.
-
Machine Learning Analysis of Electro-Coupling Mechanisms
- Paper: Haobo Li, Xinyu Li, Pengtang Wang, Zhen Zhang, Kenneth Davey, Javen Qinfeng Shi, Shi-Zhang Qiao (2024). Machine Learning Big Data Set Analysis Reveals C�CC Electro-Coupling Mechanism. Journal of the American Chemical Society.
- Outcome: Uncovered the C�CC electro-coupling mechanism using ML analysis of big data sets, advancing the understanding of chemical reactions.
-
Physics-Informed Turbulence Modeling for Particle-Laden Jets
- Paper: Xinchen Zhang, Zhen Zhang, Alfonso Chinnici, Zhiwei Sun, Javen Qinfeng Shi, Graham J. Nathan, Rey C. Chin (2024). Physics-informed data-driven unsteady Reynolds-averaged Navier�CStokes turbulence modeling for particle-laden jet flows. Physics of Fluids.
- Outcome: Introduced a physics-informed data-driven approach for turbulence modeling, improving the accuracy of simulations for particle-laden flows.
-
Non-Destructive Phenotyping of Stomatal Traits
- Paper: Phetdalaphone Pathoumthong, Zhen Zhang, Stuart J. Roy, Abdeljalil El Habti (2023). Rapid non-destructive method to phenotype stomatal traits. Plant Methods.
- Outcome: Developed a rapid, non-destructive method for phenotyping stomatal traits, enabling high-throughput analysis in plant biology.
-
Active-Learning Accelerated Catalyst Screening
- Paper: Xinyu Li, Haobo Li, Zhen Zhang, Javen Qinfeng Shi, Yan Jiao, Shi-Zhang Qiao (2023). Active-learning accelerated computational screening of A2B@NG catalysts for CO2 electrochemical reduction. Nano Energy.
- Outcome: Accelerated the discovery of efficient catalysts for CO2 electrochemical reduction using active learning, reducing computational costs and time.
Impact
The “AI for Science” project has made significant contributions to both academia and industry by:
- Enhancing the accuracy and efficiency of predictive models in engineering and fluid dynamics.
- Enabling rapid and non-destructive analysis in biological systems.
- Accelerating the discovery of new materials and catalysts for sustainable energy applications.
- Demonstrating the potential of AI to transform scientific research across disciplines.
Future Directions
- Expand Applications: Apply AI techniques to new domains, such as climate science, healthcare, and renewable energy.
- Improve Interpretability: Develop more interpretable AI models to bridge the gap between data-driven methods and domain knowledge.
- Foster Collaboration: Strengthen interdisciplinary collaborations to address grand scientific challenges.
Acknowledgments
This project is supported by Centre for Augmented Reasoning, the University of Adelaide.
Keywords
- Artificial Intelligence
- Machine Learning
- Scientific Discovery
- Predictive Modeling
- Interdisciplinary Research